Pathology Visions 2019 Poster Award winner in Best Image Analysis per DPA conference proceedings at PMC:7003557.
By: Andrew J. Schaumberg PhDa,b, Stephen Yip MD PhDc, Celina Stayerman MDd, Sanjay Mukhopadhyay MDe, and Mariam Aly PhDf,g
Affiliations: aMemorial Sloan Kettering Cancer Center and the Tri-Institutional Training Program in Computational Biology and Medicine, NY, USA
bWeill Cornell Graduate School of Medical Sciences, NY, USA
cBC Cancer, British Columbia, Canada
dLaboratorio TechniPath, San Pedro Sula, Honduras
eCleveland Clinic, Department of Pathology, OH, USA
fColumbia University, Department of Psychology, NY, USA
gAffiliate Member of the Zuckerman Mind Brain Behavior Institute, Columbia University
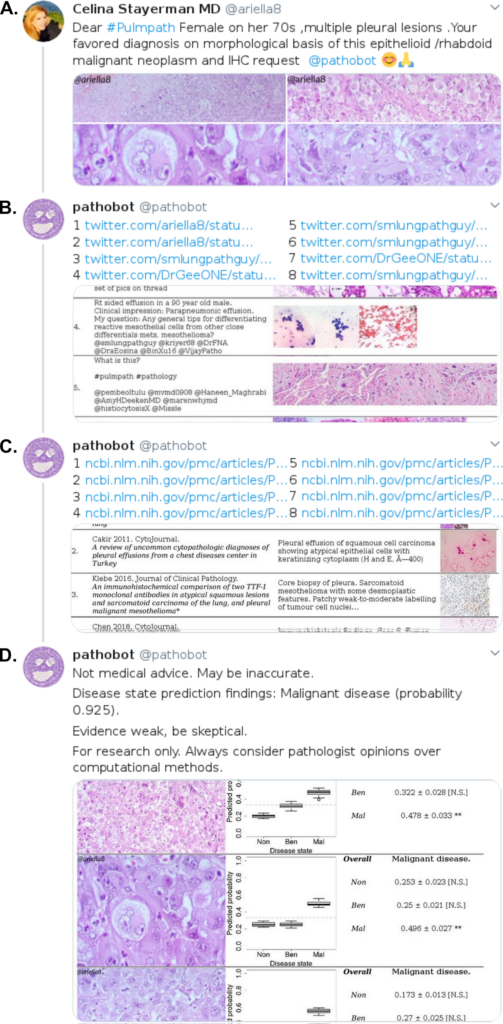
(B) Our bot (@pathobot) replies with a ranked list of potentially most similar cases on Twitter. These results show the twitter handles of the pathologists who posted similar cases in the past (e.g. @smlungpathguy and @DrGeeONE). These pathologists are notified and may be available to discuss within the Twitter app. (C) Our bot then replies to itself to show a ranked list of potentially most similar cases on PubMed. Some diseases are too rare to be in our Twitter case database, but may be in our PubMed case database. (D) Our bot again replies to itself to show a boxplot of its predicted disease state for each H&E image posted by @ariella8. Taller boxes indicate greater uncertainty in prediction. These predictions are combined into one overall prediction for the patient.
When confronted with a challenging and time-sensitive patient case, a pathologist may seek help in multiple ways. They may ask on-site colleagues for their opinions and turn to social media to ask pathologists worldwide for their thoughts. Such global access to pathologists has the potential to foster greater consensus on next steps in patient care, and improve such care worldwide.
We leveraged the power of social media and deep learning to address a simple question: who has a patient case similar to mine? In order to do that, we first assembled a team of pathologists, data scientists, computational biologists, and neuroscientists. We then turned to social media to build a database of pathology images. From Twitter, we curated 13,626 images from 6,351 tweets by 25 pathologists in 13 countries across 5 continents. To supplement this dataset with rare diseases, we added 113,161 images from 1,074,484 PubMed articles. We then applied machine learning techniques to this large-scale database in order to help pathologists find similar cases. Importantly, our method also helps pathologists connect with immediately available colleagues to discuss their cases.
In order to do this, we implemented an artificial-intelligence-driven social media bot to do “grunt work” for pathologists, e.g. search years of Twitter and PubMed history to find cases similar to the case shared by a pathologist. The search starts when a pathologist posts hematoxylin-eosin (H&E)-stained photomicrographs from their smartphone or microscope-mounted camera, along with hashtags or text descriptions (e.g., tissue type, morphological features, and/or differential diagnosis) and mentions our bot “@pathobot” in their Tweet (Fig 1A). This post activates the bot, which carries out an automated case search and returns a ranked list of the top 8 most similar cases along with the Twitter handles of the pathologists who contributed them (Fig 1B). Next, the bot replies to itself with a ranked list of the top 8 most similar cases on PubMed (Fig 1C). Our bot also predicts disease state (normal/nontumor/infection, benign/low-grade-malignant-potential, or malignant) and provides a quantitative assessment of confidence (Fig 1D). If the bot predicts the wrong disease state, a pathologist knows to disregard the search results, because the artificial intelligence method is not correctly interpreting the case. This process takes place in public view on social media, and draws in other pathologists who comment on the case and search results.
We aim to use multiple sources of feedback and experience to improve the automated bot search. For example, we encourage pathologists to repeatedly use pathobot search for the same case as more information becomes available through patient history, specialized stains, colleague opinions, etc. This allows pathobot to refine and improve the search as more information is obtained. Like any search, results may improve using different “search terms”, e.g. different H&E images or more potential diagnoses to consider in the differential. Through continual feedback and interaction with pathologists, we seek to improve our machine learning approaches so that pathologists can gain the maximum value from our tools — even though such tools will never replace the expertise of a pathologist. Rather, we hope to be able to augment pathologists’ capabilities by combining artificial intelligence, social media, and a globally distributed network of expert pathologists. This will be especially important for hospitals or regions that lack subspecialty expertise in the specific disease at hand.
We are honored that a preliminary version of our work, presented at the Digital Pathology Association’s Pathology Visions conference in 2019, won Best Poster for Image Analysis. The expanded manuscript is now online at Modern Pathology (https://www.nature.com/articles/s41379-020-0540-1). Please stay tuned to http://pathobotology.org and http://twitter.com/pathobotology for our data sharing to be announced.

In light of increased telepathology from the COVID-19 pandemic, we used our “pathobotology” platform to distribute free 3D-printed smartphone-to-microscope mounts (i.e. a “#pathobox”) for pathologists and medical students (Fig 2). Some pathologists use smartphones to share photos or video conference with each other through apps. We distributed these ‘pathoboxes’ free of charge to pathologists in developing countries, who may not have access to microscope-mounted cameras (https://twitter.com/pathobotology/status/1250762627439046656). Because some pathologists redeploy for COVID-19 patient care, we collaborated with volunteers at New York University to make 3D-printed face shields for frontline healthcare workers in New York City (https://twitter.com/i/events/1252064962182361091).
We hope to be able to use our tools to provide some help in the COVID-19 pandemic. Pathobot currently has older coronavirus-related articles in its PubMed database, often SARS or MERS (https://twitter.com/schaumberg_a/status/1243267484641263618). COVID-19 H&E images are scarce on social media, and do not exist in pathobot’s dataset. We would be grateful for the community to collaborate with us by sharing any H&E-stained photomicrographs from COVID-19 cases. We can then add these cases to pathobot’s database to help other pathologists find similar COVID-19 cases and discuss.
One challenge with using smartphones, microscope-mounted cameras, and microscopes for sharing images is that they all have a small field of view. A single photo conveys far less context than a whole slide image. To virtually widen the field of view, our pathobotology website also freely provides the “#pathopan” software tool, which stitches together adjacent microscopy photos like a panorama. Together, the pathobox and pathopan endeavors further our goal of connecting pathologists worldwide, especially those with limited resources.
We are always looking to build our research and collaborate with others. If you are interested — whether your expertise is medical or computational — please reach out to discuss collaboration, i.e. http://pathobotology.org/contact. Such collaboration will help us cover more disease entities, broaden discussion among more experts, and apply better methodology in the interest of patient care worldwide. In particular, given the imminent and widespread challenges of COVID-19, we would most welcome the sharing of COVID-19 cases to add to our pathology database. This would help other pathologists find these cases as well, and generate discussion that can help patient care across the world.
Reference
[1] Schaumberg AJ, Juarez-Nicanor WC, Choudhury SJ, Pastrián LG, Pritt BS, Prieto Pozuelo M, Sotillo Sánchez R, Ho K, Zahra N, Sener BD, Yip S, Xu B, Rao Annavarapu S, Morini A, Jones KA, Rosado-Orozco K, Mukhopadhyay S, Miguel C, Yang H, Rosen Y, Ali RH, Folaranmi OO, Gardner JM, Rusu C, Stayerman C, Gross J, Suleiman DE, Sirintrapun SJ, Aly M*, Fuchs TJ*. [* = co-PI] (2020). Interpretable multimodal deep learning for real-time pan-tissue pan-disease pathology search on social media. Modern Pathology. Available at https://doi.org/10.1038/s41379-020-0540-1. Preprint available on bioRxiv at https://doi.org/10.1101/396663.
Disclaimer: In seeking to foster discourse on a wide array of ideas, the Digital Pathology Association believes that it is important to share a range of prominent industry viewpoints. This article does not necessarily express the viewpoints of the DPA, however we view this as a valuable point with which to facilitate discussion.
Please log in to your DPA profile to submit comments